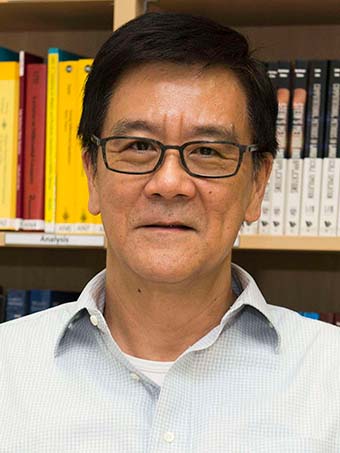
Email: phylaich@nus.edu.sg
Office: S12-02-01B
Tel: +65 6516 2981
Current Research
- The underlying physical principles of machine/deep learning (M/DL), and the relationship between the learning processes and the notion of edge of chaos
- Physics-assisted machine learning in scientific discovery – conceptual foundations, algorithms and applications
- Dynamical processes in complex systems, and the applications of complexity science in the science of cities (urban resilience, e.g.)
FIG. 2. Model evolution path in the order-chaos phase diagram and related learning curves. The optimal epoch in (a)-(c) is the epoch with the minimum test loss. (c) shows that the optimal epoch also has the almost largest test accuracy. As the value of J0 is very small during training, we only plot the order-chaos phase diagram for -1 < J0/J < 1.
Edge of chaos and deep learning
The success of deep neural networks in real-world problems has prompted many attempts to explain their training dynamics and generalization performance, but more guiding principles for the training of neural networks are still needed. Motivated by the edge of chaos principle behind the optimal performance of neural networks, we study the role of various hyperparameters in modern neural network training algorithms in terms of the order-chaos phase diagram. In particular, we study a fully analytical feedforward neural network trained on the widely adopted Fashion-MNIST dataset, and study the dynamics associated with the hyperparameters in back-propagation during the training process. We find that for the basic algorithm of stochastic gradient descent with momentum, in the range around the commonly used hyperparameter values, clear scaling relations are present with respect to the training time (epochs) during the ordered phase in the phase diagram, and the model’s optimal generalization power at the edge of chaos is similar across different training parameter combinations. In the chaotic phase, the same scaling no longer exists. The scaling allows us to choose the training parameters to achieve faster training without sacrificing performance. In addition, we find that the commonly used model regularization method – weight decay – effectively pushes the model towards the ordered phase to achieve better performance. Leveraging on this fact and the scaling relations in the other hyperparameters, we derived a principled guideline for hyperparameter determination, such that the model can achieve optimal performance by saturating it at the edge of chaos. Demonstrated on this simple neural network model and training algorithm, our work improves the understanding of neural network training dynamics, and can potentially be extended to guiding principles of more complex model architectures and algorithms.
Selected Publications
- L. Zhang, L. Feng, K. Chen and C. H. Lai, “Scaling relations of training hyper-parameters in deep learning”, Conference on Complex Systems CCS2021, Lyon, France, 25-29 October 2021.
- L. Zhang, L. Feng, K. Chen and C. H. Lai, “Edge of chaos as a guiding principle for modern neural network training”, arXiv:2107.09437 (July 2021)
- L. Zhang, L. Feng, K. Chen and C. H. Lai, “Asymptotic stability of the neural network and its generalization power”, APS March Meeting, 15-19 March 2021.
- L. Zhang, L. Feng and C. H. Lai, “Mutual information dynamics in deep learning”, Satellite Session CCS2019.
- N. N. Chung, L. Y. Chew, W. Chen, R. M. D’Souza and C. H. Lai, “Susceptible individuals drive active social contagion”, Physical Review Research 1, 033125 (2019)
- S. Ma, L. Feng and C. H. Lai, “Mechanistic modelling of viral spreading on empirical social network and popularity prediction”, Scientific Reports 8, 13126 (2018)
- H. S. Sugiarto, N. N. Chung, C. H. Lai and L. Y. Chew, “Emergence of cooperation in coupled socio-ecological system through a direct or an indirect social control mechanism”, Journal of Physics Communications 1, 055019 (2017)